This essay was originally written as part of my first-year engineering degree coursework. A typeset copy can be downloaded here.
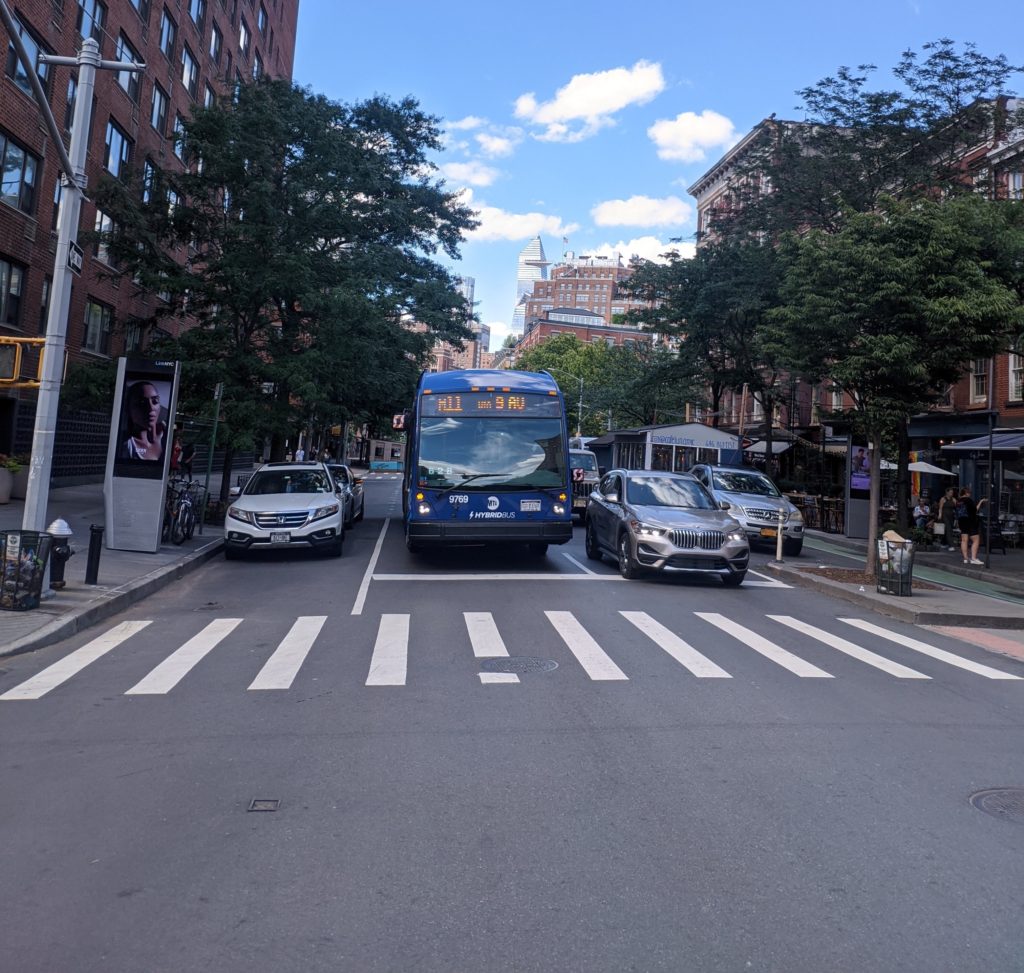
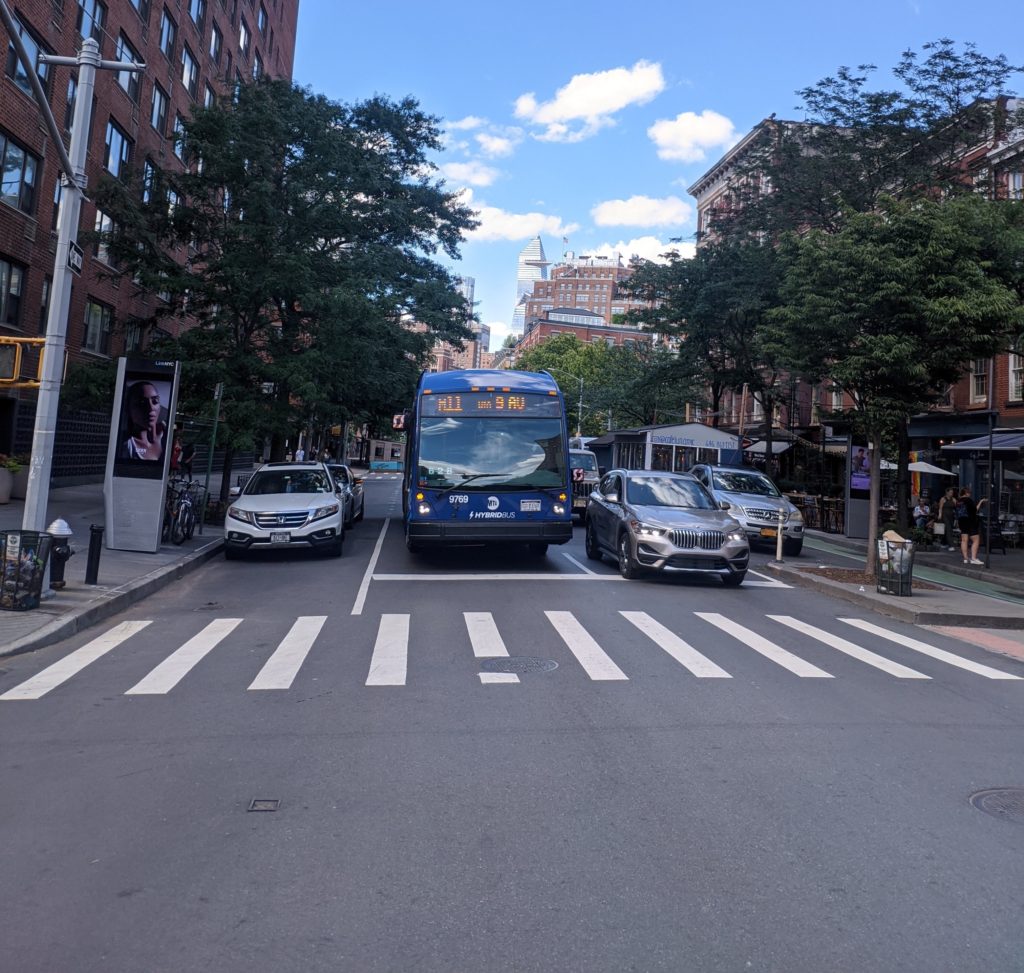
As engineers move to create machines that must make life-and-death decisions without human intervention, a whole new range of ethical challenges present themselves. From medical robots to autonomous vehicles, ethical decision-making will soon need to be programmed into control sequences. Engineers must thus confront moral dilemmas which will have potentially enormous real-world outcomes. This report will discuss these issues, specifically pertaining to self-driving cars and the ethical implications of their introduction.
First, it is useful to consider the scenarios in which ethical problems arise most clearly, namely, unavoidable crashes (Nyholm, 2018). Self-driving cars promise to be much safer than traditional cars, with an estimated 94% of crashes caused by human error (National Highway Traffic Safety Administration, 2022). Advanced sensors and algorithms significantly improve the awareness and response times of vehicles when compared to human drivers. However, when driving on roads with unpredictable non-autonomous cars, pedestrians, and cyclists, crashes are still inevitable. Where in traditional unavoidable crashes, decision making may have been left to chance or driver morality, technology in self-driving cars allows for a more advanced analysis of the surroundings to inform a reasoned response. This will lead to situations, given widespread use, in which car control systems must decide which lives to prioritise in an unavoidable collision. For these decisions to be made, an implementation of machine ethics is required: an intersection of engineering with philosophy.
What constitutes a machine acting ethically is a complex question; but insofar as machines are able to act ethically at all, we can organise them into two distinct levels of agency (Moor, 2006). Implicit ethical agents are machines that have ethical behaviour built into them. For example, engineers have designed autonomous vehicles to avoid hitting and injuring pedestrians. Explicit ethical agents, by contrast, are machines that can derive their own code of ethics based on their obligations, principles and experiences (M. Anderson and S. L. Anderson, 2007). It is unclear if such a machine yet exists or whether their implementation would even be desirable: two different machines with explicit ethical agency might reach two different conclusions in similar scenarios, and there would be no way of knowing exactly why (Deng, 2015). This unpredictability is one reason why much of the existing self-driving research favours implementing implicit ethical agency based on formulated rules.
With implicit self-driving implementations, moral agency is placed upon engineers and ethicists. They must decide the rules that govern the vehicle decision-making in critical situations: but how can they choose what is right or wrong for a diverse range of product users? One way to help answer this question might be to look at two traditional ethical theories (Nyholm, 2018) concerning the morality, intentionality and motivation behind actions.
Utilitarian ethics has a focus on the outcome of conduct. A utilitarian would argue that the right action to take would be the one that maximises happiness, regardless of intentionality (Haslanger, 2017); in the context of a self-driving collision, this might mean sacrificing the occupants of the vehicle to minimise overall loss of life. However, a utilitarian approach would also consider the effect of such a system on technology uptake. While most people agree with utilitarian rules for others, they would disapprove of driving in a car that might sacrifice their own life for the putative greater good (Bonnefon, Shariff, and Rahwan, 2016). Implementing ‘utilitarian’ rules in all self-driving cars may be counterproductive in reducing casualties (Emerging Technology from the arXiv, 2020), because it could lead to a slower reduction in human drivers, and hence avoidable accidents, on the road. As such, it could be argued that implementing rules to prioritise the driver’s safety would cause the least overall suffering, by maximising uptake.
Kantian or deontological ethics instead has a focus on principles: the intention of an action is what determines if it is right or wrong, not the consequences. Kantian thought would follow the “you are no exception” principle (Haslanger, 2017). For example, if everyone cut into a queue, the queue would move no faster, therefore cutting into the queue is wrong. Another Kantian maxim is the “respect for persons” principle, that each individual is a source of value and should never just be treated as a means to an end. An implicit self-driving control implementation could not be Kantian itself as it is not a moral agent (Gurney, 2015) so cannot have intention; however, engineers looking at the issue from a Kantian viewpoint would oppose any suggestion of prioritising one life over another, even if this would reduce overall casualties. Kantians cannot rank actions in order of merit (Haslanger, 2017); determining procedures to follow in an unavoidable crash is beyond the scope of this ethical theory.
Neither of these ethical theories are directly applicable to the rules engineers must choose for a self-driving implementation. Utilitarianism does lend itself more to situations where an abundance of information is available and definite decisions must be made. However, this ethical framework does not align with societal opinion. Most would recognise the difference between murder and failing to act to save someone; this is reflected by the former being a crime in the UK, whereas the latter is not (Gurney, 2015). It must also be considered how a utilitarian algorithm calculates the maximum utility in a crash situation. Does maximising happiness take into account the age, social status or health of the victims? Or would considering these traits be discriminatory? Beyond looking solely at ethical theories, analysis of people’s attitudes can be useful to help guide moral rules engineers implement.
The Moral Machine experiment (Awad et al., 2018) explores how people say they would react in different pedestrian crash scenarios with inevitable fatalities. The scenario variables included victim sex, age, quantity and social status; participants were also told if the pedestrians were crossing legally. Globally, the responses showed a strong preference for saving humans over pets, maximising the number of lives saved and sparing the young over the old; beyond these similarities, many of the respondents’ moral preferences varied by country (Maxmen, 2018). This inconsistency raises the question: should autonomous vehicles all have the same ethical settings? Given the geographical response differences (Awad et al., 2018) these settings could instead vary by region. Alternatively, it could be left to vehicle owners to calibrate their machine’s crash decision-making. However, implementing such a system may prevent co-operation between vehicles in unavoidable crashes and would allow owner prejudice to influence life-death decision making (Nyholm, 2018).
Engineers have a responsibility to respect life, law and the public good (Royal Academy of Engineering, 2011). To what extent they can delegate this responsibility onto non-sentient machines and how exactly their obligation is fulfilled when people and steel are moving at high speed is unclear, but will require careful consideration of the raised arguments.
References
Anderson, M. and S. L. Anderson (Dec. 2007). “Machine Ethics: Creating an Ethical Intelligent Agent”. In: AI Magazine 28.4, p. 15. doi: 10.1609/aimag.v28i4.2065.
Awad, E. et al. (2018). “The Moral Machine experiment”. In: Nature 563.7729, pp. 59–64. issn: 0028-0836. doi: 10.1038/s41586-0180637-6.
Bonnefon, J.-F., A. Shariff, and I. Rahwan (2016). “The social dilemma of autonomous vehicles”. In: Science 352.6293, pp. 1573–1576. doi: 10.1126/science.aaf2654.
Deng, B. (July 2015). “The robot’s dilemma”. In: Nature 523.7558, pp. 24–26. doi: 10.1038/523024a.
Emerging Technology from the arXiv (2020). “Why self-driving cars must be programmed to kill”. In: MIT Technology Review. url: https://www.technologyreview.com/2015/10/22/165469/whyself-driving-cars-must-be-programmed-to-kill/ (visited on 01/01/2022).
Gurney, J. K. (2015). “Crashing into the unknown: an examination of crash optimization algorithms through the two lanes of ethics and law”. In: Albany law review 79.1, p. 183. issn: 0002-4678.
Haslanger, S. (2017). “Three Moral Theories”. In: 24.03 Good Food: Ethics and Politics of Food. MIT OpenCourseWare. url: https://ocw.mit.edu/courses/linguistics-and-philosophy/24-03-good-food-ethics-and-politics-of-food-spring-2017/lecture-notes/ MIT24_03S17_lec03.pdf (visited on 01/01/2022).
Maxmen, A. (2018). “Self-driving car dilemmas reveal that moral choices are not universal”. In: Nature 562.7728, pp. 469–470. issn: 0028-0836. doi: 10.1038/d41586-018-07135-0.
Moor, J. H. (2006). “The Nature, Importance, and Difficulty of Machine Ethics”. In: IEEE intelligent systems 21.4, pp. 18–21. issn: 1541-1672. doi: 10.1109/MIS.2006.80.
National Highway Traffic Safety Administration (2022). Automated vehicles for safety. url: https://www.nhtsa.gov/technology-innovation/automated-vehicles-safety (visited on 01/01/2022).
Nyholm, S. (2018). “The ethics of crashes with self-driving cars: A roadmap, I”. In: Philosophy Compass 13.7, e12507. doi: 10.1111/phc3.12507.
Royal Academy of Engineering (2011). Engineering ethics in practice: a guide for engineers. London: Royal Academy of Engineering. isbn: 190349673X.